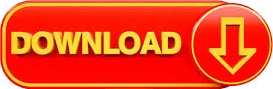
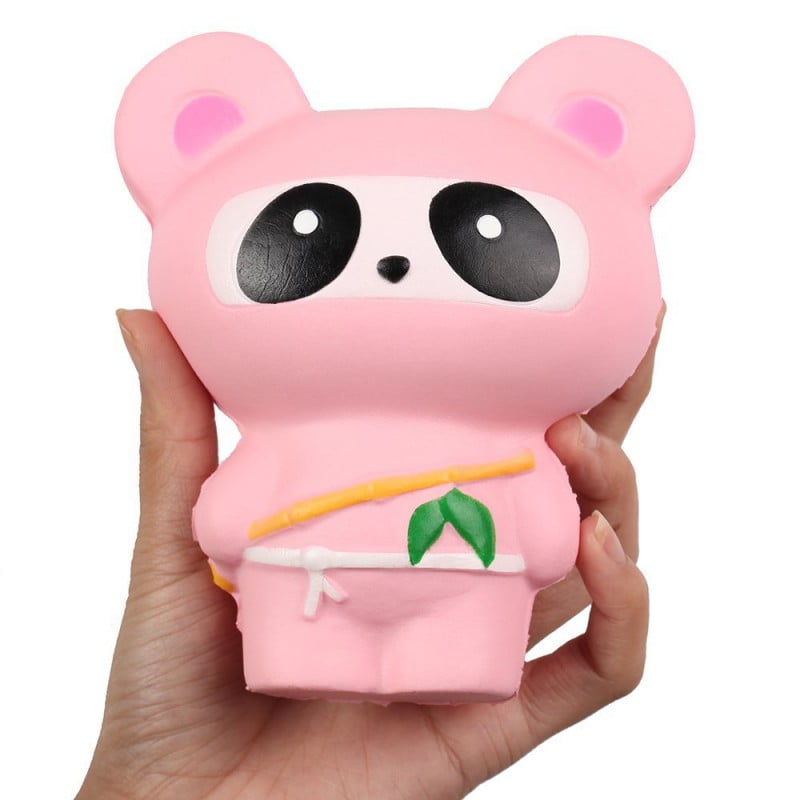
In fact, the process starts with the positions estimation using previous proposed methods based on RT by exploiting our optimized channel model. Thus, we propose an original method that combines RT based localization method with the Bayesian filtering approach. In this paper, the problematic of moving target is addressed.

It has attracted more and more attention for the solution of localization problems, , employing learning methods like support vector machines (SVMs), neural networks (NNs), k-nearest neighbor as well as fuzzy logic, which proved their suitability in the understanding of the complex relations between the RSSI behavior and the target position. Machine learning is the field of the artificial intelligence that deals with the design and the development of algorithms and techniques which allow the system to learn the rules and the models from data. Thus, researchers have been motivated to develop improved solutions to enhance efficiency and accuracy of positioning. Thus, the main challenge in localization is to reduce the RSSI fluctuations. However, signal propagation inside buildings is affected by many environmental constraints such as reflections, interference, pathloss fading and shadowing. Among the available solutions for indoor localization, the ones based on the received signal strength indicator (RSSI), have been widely investigated since the RSSI is available in most of the commercial wireless devices without the need of any additional sensors or hardware customization. Accordingly, the development of novel methods of localization has became a great concern for the wireless sensor networks. The main constraint of these techniques is the high cost since they require extra hardware to measure such parameters. Among the range based parameters, we find the angle of arrival (AOA), the time difference of arrival (TDOA), the ultra-wideband (UWB) frequency spectrum. Range based localization methods are widely investigated in the literature. Alternative positioning and tracking solution using WSNs have been proposed. Global positioning system (GPS) is one of the well-known solutions to the localization problem, but its application to indoor environments is still an open issue. In fact, the developed localization solution should be able to provide a tradeoff between accuracy, robustness and complexity. Node position determination is critical since it is important to take into consideration many criteria. Among the significant area of research, the localization which presents an important challenge in various applications using WSN such as disasters monitoring, environment control, smart building, , agriculture, logistics and transport. This interest is expected to grow further with the high evolution of application for wireless systems.
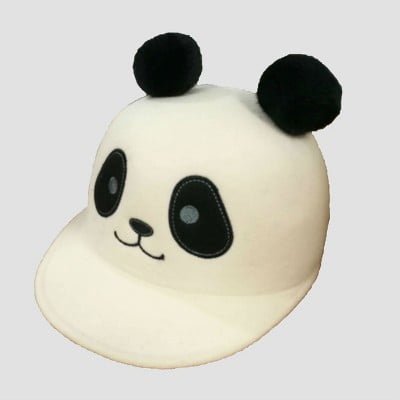
Wireless sensor networks (WSN) have attracted more and more attention in the last few years. The numerical and experimental results show the relevance and the efficiency of our method. We also compare our method to existing algorithms available in the literature. The suggested approach is evaluated through simulations and experiments. This method is combined to filtering approaches yielding to more refined results. Regression Tree algorithm is investigated in order to estimate the position using the RSSI. In this paper, we propose an innovative target tracking algorithm which combines learning regression tree approach and filtering methods using RSSI metric. Tracking using Received Signal Strength Indicator (RSSI) has been frequently adopted thanks to the availability and the low cost of this parameter. Particularly, accurate localization of a moving target is a fundamental requirement in several Machine to Machine monitoring applications. With the large use of wireless sensor devices, the interest in positioning and tracking by means of wireless sensor networks is expected to grow further.
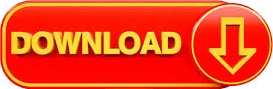